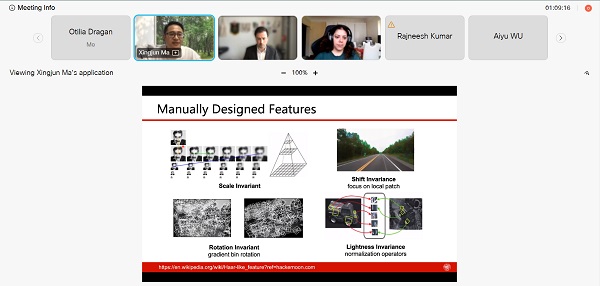
On Thursday 13 April 2023, Professor Xingjun Ma, an associate professor from Fudan University in China, spoke at an online conference on artificial intelligence (AI) and large models, organised by the Confucius Institute at the University of Luxembourg.
The conference aimed to explore new models of AI and give an overview of its history as well as some insights into possibilities for its emerging intelligence. The central question was: “Are we close to Artificial General Intelligence?” About 40 people attended online.
Professor Xingjun Ma, whose main research areas are deep learning and computer vision, explained that nowadays AI is everywhere. It has been trained and deployed to help in different fields such as autonomous driving, fintech, biology and infrastructure, to name a few.
One of the first big breakthroughs concerning AI happened in 2015, when a neural network for image recognition (ResNet) achieved a reported human-level performance on large scale image recognition. This model scored 4.94% on a top-5 test error, compared to the 5.1% human performance. This technology allows for highly accurate million-scale face recognition. This makes lip-reading technology possible, leading to potential large-scale visual speech learning.
Another big breakthrough was the use of AI for strategic games, such as Go playing. It took three weeks to train the AlphaGo model and it was able to beat both European and World Go champions in best of five matches.
A third notable AI software programme was AlphaFold, a protein structure prediction database containing 200 million structures and almost one million species. Made by DeepMind, it has reportedly been used to predict structures of proteins of SARS-CoV-2, the causative agent of COVID-19.
Currently, we find ourselves in the era of Large Models, which started in 2022 when it became possible to train extremely large models on very large amounts of web data and generalise complex tasks such as language translation, question-answering, image recognition, etc.
AI technology started in the 1970s to 1980s with the so-called “Expert System”, when certain computer programmes were able to simulate a set of fixed rules to facilitate decision-making in specific domains. “Hand-crafted features” from the 1990s to 2010s enabled the training of machine learning on manually designed features. From 2012 until 2022, deep neural networks were first used to model and solve complex problems in areas such as image recognition, natural language processing and speech recognition.
In the past, computing was understood to be the sole task of a computer. General intelligence concerns multi-tasking, the capacity for polyvalence. Contemporary systems now have multiple uses. Language models, with ChatGPT as probably the “best” one out there, according to Professor Xingjun, can be used for translation, give accurate answers, de-bug code and be used for questions and answers pertaining to human life. ChatGPT is a compilation of web pages, web documents, roughly 300 billion words.
Professor Xingjun noted that some problems of large models are, for instance, the generation of fake images and pornographic images, data leakage and copyright violations. Artists in particular have filed lawsuits because their styles and images had been borrowed, and AI-generated content could be defined as “stolen”. Privacy leakage is also an issue, for one because large models are reportedly highly efficient at facial recognition. Moreover, there may be dangers of leaks of sensitive information due to language models potentially memorising names, phone numbers, emails or even social security numbers. Professor Xingjun warned that even talking to ChatGPT can be a risk for data leakage, because questions may provide information about one’s company, emotions, problems, location and more. Users need to learn to ask the right questions. Another problem with language models is the potential for factual errors: sometimes they may produce a mix of actual information and misinformation.
Professor Xingjun’s perspective was that AI systems, while highly capable of solving problems, are still only databases. “They compare data, compress it and prompt it out,” he explained. The bigger the models, the more accurate the output information, but these large models do not have intelligence. More experiments are necessary to prove his perspective, but he admitted that sometimes when a question is answered “very cleverly”, he does consider the opposite opinion.
Humans, too, compile and compress information into easy conclusions (likes and dislikes, etc.) and carefully compute which words to use one after the other to best express their meaning and perhaps provoke admiration or understanding in their interlocutor. “We try to find logic behind everything, even where there is none,” he noted. He expressed the belief that, in some ways, humans are like large language models, and added that “we are not far from bigger findings, hopefully they can help us understand the human brain.”